The study demonstrates that AI can understand the spatial interactions between cells in tissues and extract slight information that was previously unavailable to humans. A major step forward in the use of AI for the expected progression of the disease and individualized treatment plans was achieved when researchers created an AI model that reliably forecasts results for cancer patients using tissue samples.
According to the researchers, cell spatial organization is similar to a sophisticated jigsaw puzzle in which each cell functions as a unique piece that must be precisely placed together to make a coherent tissue or organ structure.
Nature Communications details the novel method, which examines “the spatial arrangement of cells in tissue samples.” The researchers compared cell spatial organization to a complicated jigsaw puzzle, in which each cell is an individual component that must be carefully assembled to create a whole organ or tissue.
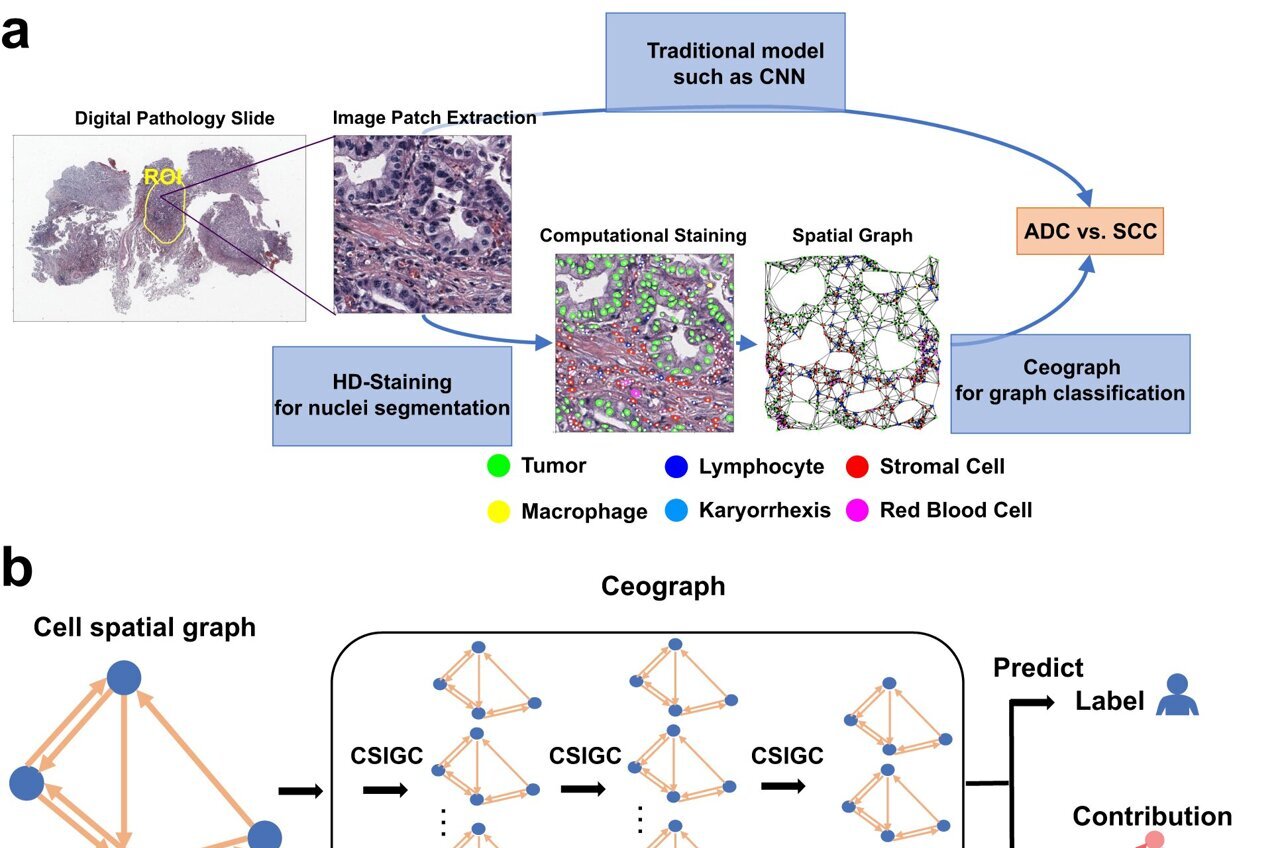
“The study showcases the remarkable ability of AI to grasp these intricate spatial relationships among cells within tissues, extracting subtle information previously beyond human comprehension while predicting patient outcomes,” stated Guanghua Xiao, a professor of the University of Texas Southwestern Medical Center in the US, who organized the research.
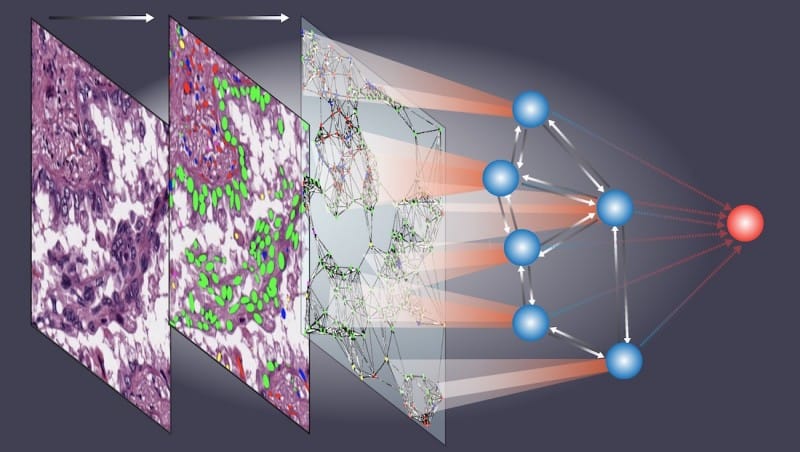
Regularly, pathologists collect patient tissue samples, mount them on slides, and examine them to arrive at a diagnosis. But, as the researchers pointed out, this process can be time-consuming, and pathologists’ judgments can differ. They also noted that humans aren’t always good at recognizing minor details in pathology images, which might be a major red flag for diagnosing a patient’s illness.
Several AI models developed in the last several years can mimic the work of pathologists in certain respects, such as classifying cells according to their type or determining the nature of connections between cells based on their proximity to one another.
However, these models fall short regarding more intricate parts of pathologists’ tissue image interpretation processes, like identifying patterns in cell spatial organization and eliminating “noise” that might confuse interpretations.
Ceograph, a novel artificial intelligence model, begins by identifying cells in pictures and their locations, like pathologists examining tissue slides. Starting with identifying cell types, it then creates a map that analyzes cell distribution, interactions, and layout based on their morphology and spatial distribution. Using pathology slides, the researchers were able to apply this approach to three different clinical circumstances effectively.
- One study employed a Ceograph to differentiate between “adenocarcinoma and squamous cell carcinoma,” two kinds of lung cancer.
- Another one involved making predictions about the progression of “precancerous lesions of the mouth,” which are oral illnesses that could be cancerous.
- The third step was determining which patients with lung cancer had the best chance of responding to a specific class of drugs known as EGF receptor inhibitors.
The Ceograph model proved far more accurate in forecasting patient outcomes than conventional approaches in every single case.
According to Xiao, the interpretable aspects of cell spatial organization found by Ceograph provide biological insights into how changes in individual cell-cell spatial interactions could have various functional repercussions. He went on to say that these results show how AI is becoming more important in healthcare and how it can help with pathology analysis by making them more efficient and accurate.”This method has the potential to streamline targeted preventive measures for high-risk populations and optimize treatment selection for individual patients,” said Xiao.