AI models have made an unexpected discovery with access to vast amounts of gene and cell data. Where will this take us? A French physician by the name of Francois-Gilbert Viault descended an Andean Mountain in 1889, extracted blood from his arm, and examined it under a microscope. The oxygen-carrying red blood cells in Dr. Viault had increased by 42%. He had unearthed a secret ability of the human body: the ability to produce more of these vital cells on demand.
A hormone was proposed as the culprit in the 1900s by scientists. The hypothetical hormone was given the Greek name erythropoietin, which means “red maker.” It took scientists seven decades and 670 liters of urine to finally isolate erythropoietin.
And around half a century later, Israeli biologists reported that they had discovered a rare type of kidney cell that produces the hormone in response to low oxygen levels. The Norn cell derives its name from the belief that human destiny was under the dominion of the Norse deities.
Finding Norn cells took 134 years for scientists. In just six weeks last summer, Californian computers found them on their own using AI models.
When Stanford researchers used AI models and taught computers to learn biology on their own, they made the finding. The machines were running an AI program like the ChatGPT, the well-known chatbot that learned a language by reading billions of text articles on the internet. However, the computers used by the Stanford researchers were educated using unprocessed data regarding the chemical and genetic composition of millions of actual cells.
The researchers did not tell the computers the significance of these measurements. They failed to mention that various cell types have distinct biological characteristics. They did not specify which cells produce antibodies or which ones, for example, capture light in our eyes.
The computers processed the data independently, building a multidimensional, massive model of every cell based on how similar they were to one another. The machines had learned an incredible lot by the time they were finished. They were able to identify more than 1,000 distinct types of cells, including ones they hadn’t seen before. The Norn cell was one of those.
“That’s remarkable, because nobody ever told the model that a Norn cell exists in the kidney,” said Stanford computer scientist Jure Leskovec, who also trained the computers.
The software is among a collection of foundation models, which are novel A.I.-powered applications focusing on the basics of biology. The models are doing more than just organizing the data that biologists are gathering. They are learning new things about the development of cells and how genes function.
Scientists believe that as the models get larger and require more computing power and laboratory data, they will begin to make deeper findings. They might hold the key to understanding cancer and other illnesses. They might discover formulas for transforming one type of cell into another.
“A vital discovery about biology that otherwise would not have been made by the biologists — I think we’re going to see that at some point,” said Scripps Research Translational Institute head Dr. Eric Topol.
It’s debatable how far they can push things. Although some doubters fear the models will eventually break down, some upbeat scientists think foundation models might even address the most important biological question of all: What differentiates nonlife from life?
For a long time, biologists have been trying to figure out how our bodies’ many cells use our DNA to execute all the various tasks that keep us alive. Industrial-scale efforts were initiated about ten years ago by researchers to extract genetic fragments from individual cells. They documented their findings in “cell atlases,” or catalogs, which grew to contain billions of data points.
Boston Children’s Hospital resident physician Dr. Christina Theodoris learned about a novel type of AI model created by Google developers in 2017 for language translations while reading about it. The model was given millions of English sentences by the researchers, along with their translations into French and German. The model gained the ability to interpret sentences that it had never encountered before. Dr. Theodoris questioned if a comparable model could learn to interpret the information found in cell atlases on its own.
She had a difficult time in 2021 locating a lab where she might attempt to construct one. “Many people didn’t think this strategy would work,” she remarked.
She was given a try by Shirley Liu, a computational scientist at the Dana-Farber Cancer Institute in Boston. Dr. Theodoris fed 30 million cells—the total amount of data from 106 previous human studies—into a program she developed called GeneFormer.
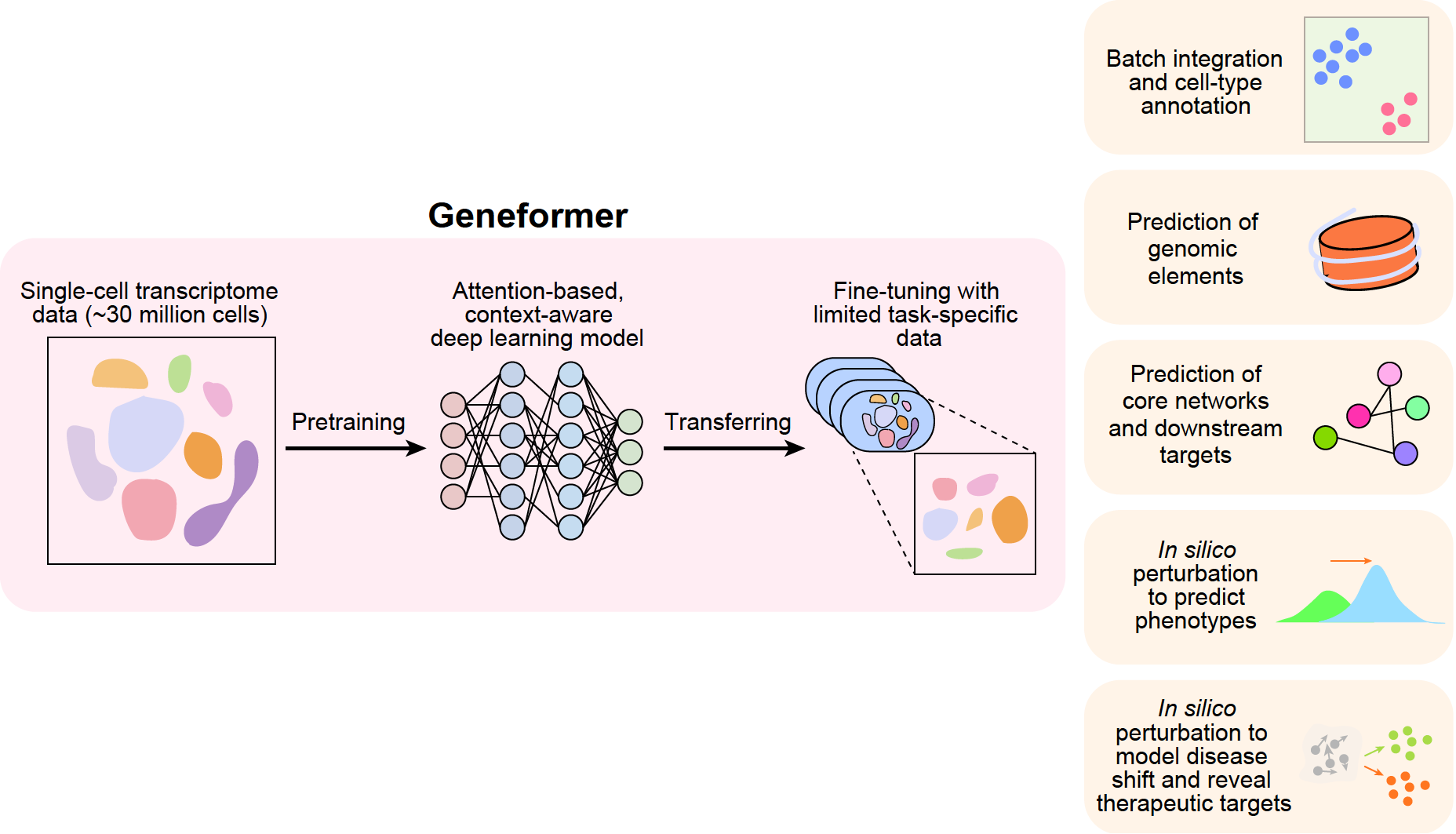
Thanks to the model, we now know a lot about how our genes act in various cell types. For instance, it foretold that a certain type of cardiac cell would be badly disrupted when a gene called TEAD4 was shut down. The prediction was tested in actual cells called cardiomyocytes by her team, and the results showed that the heart cells’ beating became weaker.
She and her colleagues conducted an additional experiment in which they exposed AI model GeneFormer, cardiac cells from both healthy and faulty individuals. “Then we said, Now tell us what changes we need to happen to the unhealthy cells to make them healthy,” explained Dr. Theodoris, who is now a computational biologist at the Gladstone Institutes in San Francisco.
GeneFormer suggested lowering levels of four genes that were never associated with cardiovascular disease before. The model advised Dr. Theodoris’s team to knock down all four genes, so they did just that. The therapy enhanced cell contraction in two of the four instances.
After contributing to the creation of CellXGene, one of the world’s largest databases of cells, the Stanford team entered the foundation-model industry. Scientists began utilizing the 33 million cells stored in the database to train their computers in August. They focused on messenger RNA, a sort of genetic information. Proteins are the end result of genetic engineering, and they also provide the model of their three-dimensional structures.
Machines have figured out ways to categorize more than a thousand different cell kinds according to the on/off regulation of their genes. You can see the clustering of 36 million cells on this map.
The model, called UCE (Universal Cell Embedding), used this data to determine how similar the cells were and then categorized them into over a thousand clusters based on their gene usage. Each grouping represented a different kind of cell that had been identified by various biologists over the years.
The process of cell division from a fertilized egg is something that UCE also taught itself. As an example, UCE found that all cells may be categorized according to the embryonic layer from which they originated. “It effectively found developmental biology,” remarked Stephen Quake, a Stanford biophysicist and co-developer of UCE.
Additionally, the model was successful in teaching itself new species. UCE was able to recognize numerous cell types in an unfamiliar animal’s genomic profile, such as a naked mole rat.
“You can bring a completely new organism — chicken, frog, fish, whatever — you can put it in, and you will get something useful out,” Dr. Leskovec added.
Following the Norn cells’ discovery at UCE, Dr. Leskovec and colleagues searched the CellXGene database to find out their origin. The kidneys were the primary source for many of the cells, although the lungs and other organs also contributed. The scientists speculated that the body may include Norn cells that had never been seen before.
University of Pennsylvania physician-scientist Dr. Katalin Susztak, who investigates Norn cells, expressed her curiosity about the discovery. “I really need to examine these cells,” she stated.
Given the lack of evidence for the erythropoietin hormone in other organs, she raises doubts about the model’s finding of genuine Norn cells outside of the kidneys. Yet, the new cells may be able to detect oxygen in the same way that Norn cells do. This means that UCE might have found a new kind of cell before scientists did.
Similar to ChatGPT, biological models do make mistakes. Computational biologist Kasia Kedzierska of the University of Oxford and colleagues recently ran a battery of experiments on GeneFormer along with another foundation model, scGPT. They gave the models unfamiliar cell atlases and asked them to do things like sort the cells into different categories. The models outperformed simpler computer programs on some tasks while failing miserably on others.
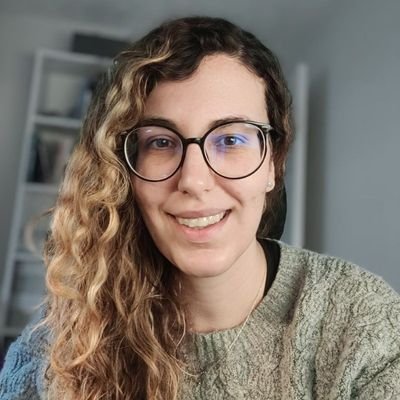
Despite her high expectations for the models, Dr. Kedzierska cautioned that “they should not be used out of the box without a proper understanding of their limitations” at this time.
According to Dr. Leskovec, the models are getting better as researchers feed them more data to train them. The most recent cell atlases provide a small quantity of data, however, when contrasted with ChatGPT’s training over the entire internet. “I would prefer a network of interconnected cells,” he expressed.
The arrival of larger cell atlases promises well for the future of cell research. Additionally, researchers are extracting various types of information from each cell in these atlases. Some researchers are collecting pictures of cells to show where proteins are located, while others are cataloging the chemicals that bind to genes. Foundational models will be able to learn how cells function with all that data.
Additionally, scientists are working on methods to enable foundation models to integrate their independent learning with the findings of traditional, human-based biologists. The plan is to link cell measurement databases with the results of thousands of scholarly articles.
It is believed that scientists would be able to construct a comprehensive mathematical model of a cell given sufficient data and computational capability.
scGPT’s developer, computational biologist Bo Wang of the University of Toronto, predicted that this development would have far-reaching, ground-breaking effects on the biological sciences. In any given scenario, he reasoned, this virtual cell might simulate a genuine cell’s behavior. Instead of using Petri dishes, scientists may conduct whole experiments on computers.
Foundation models, according to Dr. Quake’s suspicions, will discover not only the types of cells that exist in our bodies at the present time but also the kinds of cells that might exist in the future. He theorizes that specific biochemical combinations are required for cellular survival. In his ideal world, Dr. Quake would use foundational models to create a map of the possible realm, the boundary beyond which life would be impossible.

Doctor Quake expressed his belief that these models will aid in gaining a basic understanding of the cell, that will provide insight into the nature of life.
Also, if scientists have a good idea of what can and cannot support life, they may be able to make cells that don’t exist in nature. Chemical formulas that turn common cells into unique, exceptional ones may be manufactured by the foundation model. A damaged organ may be explored, and its condition reported by those new cells or plaque in blood vessels could be destroyed.
“It’s reminiscent of ‘Fantastic Voyage,’” Dr. Quake confessed. “However, the future’s course is completely unpredictable.”
There will be many additional dangers if the foundation models are as good as Dr. Quake had hoped. Eighty biologists and AI professionals have signed a petition demanding regulation of the technology to prevent the development of new biological weapons. The petition was submitted on Friday. This worry could extend to novel cell types generated by the simulations.
Even sooner, there might be privacy breaches. The goal of the research is to develop individualized models of the foundation that take into account each person’s genome and how it functions in cells. This additional layer of information may provide light on the effects of various gene variants on cellular processes. On the other hand, it has the potential to provide the foundation model’s owners with unprecedented access to personal details about the individuals whose DNA and cells were contributed to scientific research.
The extent to which basic models will progress towards “Fantastic Voyage,” though, is a matter of some uncertainty among scientists. Data quality determines how accurate the models are. The ability to gather data that we haven’t yet mastered might be crucial to making a ground-breaking discovery on life. Data requirements for the models may be unknown to us.
Arizona State University physicist Sara Walker, who investigates the molecular underpinnings of life, speculated that “they might make some new discoveries of interest.” “However, in the end, they have severe limitations when it comes to ground-breaking new discoveries.”
Nonetheless, foundation models’ results have already made their developers question what humans would do in a future where computers can solve complex biological problems without human input. Research into the mechanisms of life has traditionally been recognized and rewarded for the innovative and labor-intensive experiments conducted by biologists. Computers, however, may be able to scan billions of cells for patterns that humans can’t perceive in a few days, weeks, or even hours, revealing these inner workings.
“It’s going to force a complete rethink of what we consider creativity,” Dr. Quake said. “Professors should be very, very nervous,” Dr. Quake warned.